Public Interest Tech Principles Can Shape the Future
of Data Science and Artificial Intelligence
Data Science & AI
May, 2023
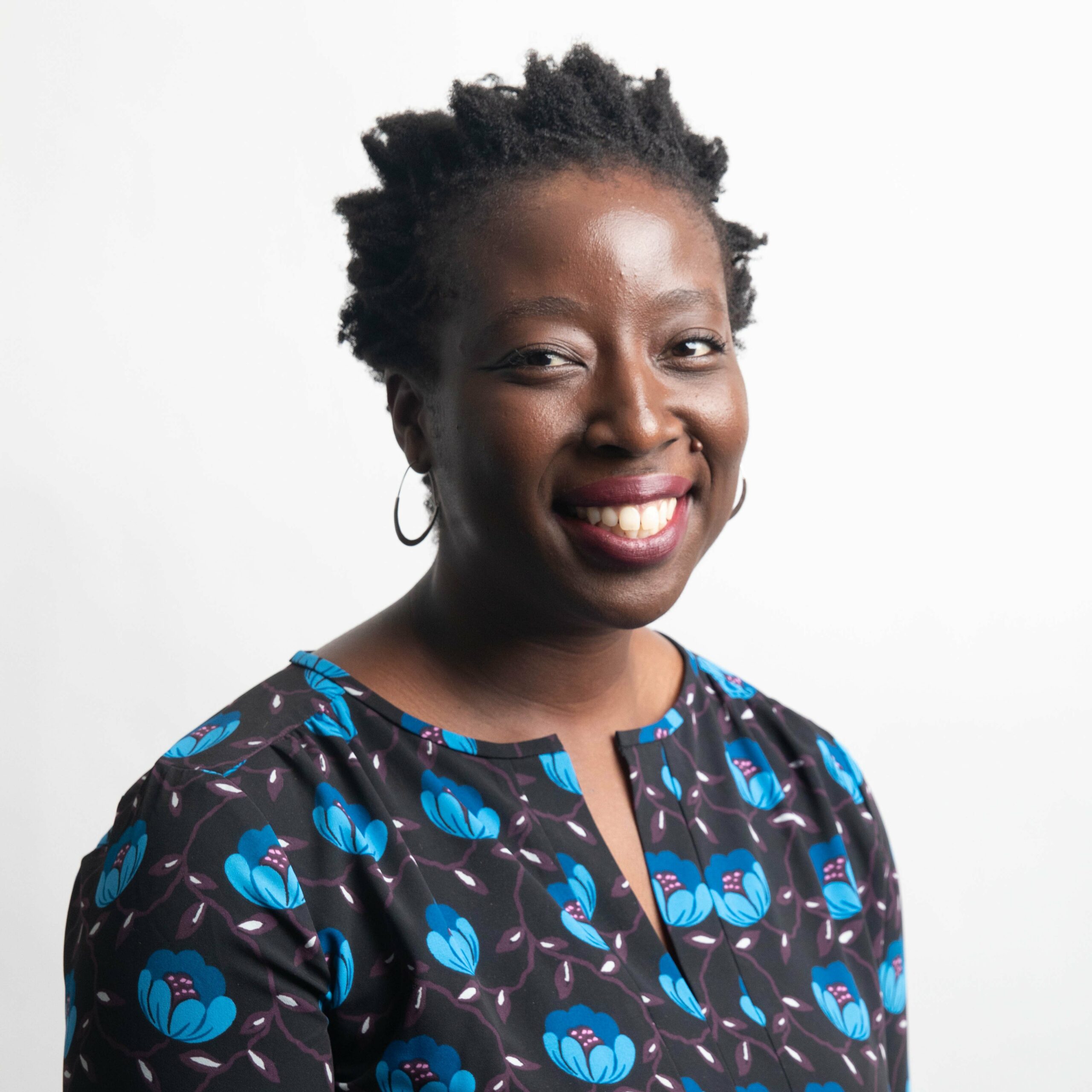
Author: Afua Bruce is the founder of the ANB Advisory Group, co-author of The Tech That Comes Next and former Director of Engineering at New America Public Interest Technology. In early 2023, ANB Advisory Group conducted a scan of data science for impact programs at PIT-UN member institutions, and also conducted a review of data science projects that have received PIT-UN Challenge funding.
It has been more than a decade since Harvard Business Review declared the profession of data scientist to be the “sexiest job of the century.” Since then, we have seen industry embrace data science as businesses seek ways to differentiate themselves using insights and predictions based on data of their consumers, their markets, and their own organizations. Accordingly, research into data science has increased, and academic institutions have created a number of credentialed programs and research institutes for students and faculty. Data science’s ability to positively impact the speed of operations and efficiency of organizations has been proven. However, as many scholars, practitioners, and advocates have pointed out, that same speed and efficiency can also magnify social inequities and public harms.
At the same time, the field of artificial intelligence has greatly expanded, as has its embrace by industry and the general public. AI now streamlines how organizations take notes, process payroll , recommend products to clients, and much, much more. Recent product releases and headlines about artificial general intelligence (the theoretical possibility that AI could perform any task humans can perform) have spurred a new round of conversations about how AI could transform human society — or destroy it altogether, depending on one’s perspective.
With widespread use of AI, the workforce will certainly shift as some tasks and perhaps even entire jobs will be performed by AI systems. Many colleges have made significant investments in AI research programs. Many institutions have recognized the importance of training students in how to design and develop AI systems, as well as how to operate in a world where AI is prevalent. And once again, many scholars, practitioners, and advocates have warned that without more intentional and ethical designs, AI systems will harm, erase, or exclude marginalized populations.
The Intersection of Data Science, AI and Public Interest Technology
Data science and artificial intelligence are two separate, but related, computational fields. As Rice University’s Computer Science department describes:
While there is debate about the definitions of data science vs. artificial intelligence, AI is a sub-discipline of computer science focused on building computers with flexible intelligence capable of solving complex problems using data, learning from those solutions, and making replicable decisions at scale.
Data scientists contribute to the growth and development of AI. They create algorithms designed to learn patterns and correlations from data, which AI can use to create predictive models that generate insight from data. Data scientists also use AI as a tool to understand data and inform business decision-making.
Practically, at some institutions, data science and artificial intelligence programs are sometimes seen as competitors for talent and funding, sometimes seen as collaborators, and sometimes remain organizationally separate. As both data science and artificial intelligence garner more and more attention from universities, students, and employers, we must ask ourselves how to balance the promise and excitement of these fields with the need to develop the associated algorithms responsibly. When systems can automatically have an impact on who is eligible to be hired or promoted, who gets access to housing, or who can receive medical treatments, those designing the systems must understand how to approach problems with not just efficiency and profitability in mind, but also equity, justice and the public good.
Public interest technology provides a framework to tackle these challenges. “By deliberately aiming to protect and secure our collective need for justice, dignity, and autonomy, PIT asks us to consider the values and codes of conduct that bind us together as a society,” reads an excerpt from PIT-UN’s core documents.
What could it mean for designers and implementers of data science and AI to “advance the public interest in a way that generates public benefits and promotes the public good”? Public interest technology provides a way to ask, research, and address the following key questions:
- How do technologists ensure the tools they design are deployed and governed responsibly within business, government, and wider societal contexts?
- What data sets and training data are being used to design these systems? Do they represent the nuance of human populations and lived experience? Are they representative enough to ensure that analyses or predictions based on the data will be fair and just?
- How do decisions made early in the data science life cycle affect the ultimate efficacy and responsiveness of systems?
- How will acceptable accuracy rates be determined for different applications?
- Are there ways to turn on and off the algorithms as needed?
- What accountability structures and auditing systems can be built to ensure the fairness of data science and AI algorithms across industries?
Examples of Public Interest Data Science and AI
Over the past several years, an increasing number of academic institutions have recognized the importance of applying data science and AI in the public interest and have created extracurricular clubs, classroom and experiential courses, and certificate and degree programs that train students to consider how data science and AI affect communities in different ways and how these tools can be designed and deployed in new, beneficial ways.
A field scan by the ANB Advisory Group shows that students at PIT-UN institutions are learning vital historical context, working on interdisciplinary teams, and translating data insights into language that policymakers, community organizations, and businesses can understand.
For example, in Boston University’s BU Spark!, five program staff assign students to teams and manage semester-to-semester relationships with government agencies and nonprofit organizations. Students have used data science to conduct sentiment analysis of Twitter feeds for a national civil rights organization and regularly provide data analysis for the Boston City Council. Over 3,000 students have learned how to work with real-world, messy data, and how solving data problems can contribute to solving larger organizational or societal problems. In addition to technical courses, students learn critical sociological skills, such as how to understand race in the context of using census data. BU Spark! is one of many programs throughout PIT-UN members demonstrating that labs (including summer programs and practical courses) are an effective way for students to learn public interest tech ideas in real-world contexts and to practice co-design and co-development with affected community partners.
Penn State’s “AI for Good, Experiential Learning & Innovation for PIT” program was one of a handful of PIT-UN grantees to train both college students and working professionals in the ethics and techniques of artificial intelligence. The program developed a new slate of experiential learning opportunities for college students, along with an online microcredential course for professionals in any sector. While it is important to train the next generation of technologists, we must also consider how to train today’s leaders and decision makers.
Similarly, Carnegie Mellon University launched a Public Interest Technology Certificate program in 2022. Geared toward employees in all levels of government, the six-month program trained its first cohort in data management, digital innovation, and AI leadership “to create a more efficient, transparent, and inclusive government.” Training mid-career professionals while also building a PIT network that can inform and support their work can lead to real-world impact well beyond the walls of the university.
Key Lessons & Recommendations
These are just two of the many projects across PIT-UN applying a public interest framework to data science and AI challenges. And universities can do even more. Although the development and use of data science and AI differ, some of the application settings and opportunities to affect change have similar underlying challenges. Therefore, the following three recommendations can apply to both data science and AI programs.
As federal, state, and local policies and initiatives encourage the advancement of data, government agencies will seek not just support in accessing data, but also access to advanced data science tools to make data actionable. Miami Dade College, for example, worked with the nonprofit Code for South Florida, Microsoft, and the city of Miami to create a participatory web app that helps Miami residents become informed contributors to the city’s budget. In their 2019-2020 PIT-UN Challenge project, MDC created a GIS certificate course for underrepresented students to contribute to mapping the impacts of climate change.
Using data science to make clear policy recommendations or create policy products — especially in collaboration with other stakeholders — is a great way to provide students with experiential learning opportunities while also increasing the reach and impact of public interest tech’s core ideas.
2. Define PIT competencies for data science and AI
As colleges and universities create and expand both data science programs and AI, both students and professors seek courses grounded in strong research and clear outcomes. Projects such as Georgia State’s Public Interest Data Literacy Initiatives have created individual courses that offer PIT frameworks for data science and AI. We are at a point where PIT-UN schools could collaborate to create an inclusive set of standard competencies. Such standardization could lend more credence and visibility to PIT degrees and could be a prototype for standards required of all data science and AI practitioners regardless of sector.
Students — and even faculty — seek practical experience that they can put on their resumes, describe to potential employers, and use to forge cross-sector partnerships. PIT-UN has consistently funded experiential learning projects to strengthen the pipeline of technologists who understand how to apply data science and AI in the public interest.
Columbia University and Lehman College’s Public Interest Technology Data Science Corps placed college students in teams to use data science to support New York City agency projects to improve the lives of local residents. Ohio State University placed PIT fellows in state government to encourage young technologists to consider public service, while fostering a culture of collaboration between the public sector and academia. These are just two examples of how meaningful internships and experiential learning speak to the interests of students and faculty while growing PIT’s public reputation.
Our Task Going Forward
The sustained interest in and excitement about both data science and artificial intelligence bodes well for the future of academic programs dedicated to these concepts. More significantly, the ways in which industries and community organizations operate will change and be changed because of advancements with these technologies.
Making these changes more positive than negative, and actively reducing adverse disparities, will require sustained work, new ways of training practitioners, and usable recommendations and tools to shape a more just technology ecosystem. Public interest technology’s emphasis on equity and justice provides the necessary lens to guide the development and use of these technologies. As PIT-UN Program Manager Brenda Mora Perea reminds us, it is our job to keep these concepts at the center of all we do and to advocate for social responsibility at every stage of technology design, deployment, and governance.